How 11x Built Deep Research Agents with Letta
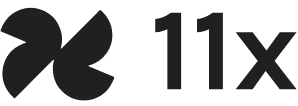
Letta is so easy to understand. Anyone can do it. The Agent Development Environment [ADE] is the Cursor of agent building.
How 11x Delivers Deep Research Agents for Every Customer Using Letta
In just 72 hours, 11x transformed their AI-powered sales automation platform by building a Deep Research agent on Letta. Their journey reveals how the right tools can rapidly speed up iteration speed and time to production for AI agents, and also unlock entirely new product possibilities.
The Challenge: Context Windows and Customer Trust
"We're hitting context limits" - Keith Fearon, Head of Growth at 11x, faced a problem familiar to many AI-first companies. Their B2B sales automation platform relied on structured "offers" that customers would manually create, containing pain points, solutions, and proof points to inform automated messaging.
This approach worked initially, but quickly hit limitations. "Basically, our customers want to train a mini human SDR using AI so they give us all their data," explained Keith. As customers added more products and services—sometimes hundreds—they would quickly exceed token limits when trying to add all the necessary context into their prompts.
The team built a RAG system as an obvious solution, but this introduced a new problem: trust. "People don't know what info the AI is looking at and why," Fearon continued. "People don't have any trust in that sort of AI system, with our very non-technical end users."
For a product positioning itself as a "digital worker" rather than just software, this lack of transparency became a critical barrier. Customers couldn't see what information was being used from their knowledge base, and with a standard RAG setup it was also very hard to explain what kind of data the LLM was seeing, and why, leading to hesitation about using the AI features.
"By default, when you create a campaign on our platform, all offers are selected," explained Prabhav Jain, 11x’s CTO. "Imagine we have a large customer that adds 200 offers to our platform. That's when your context limit completely blows up." The impact of these limitations was clear: despite the potential of their AI SDR agents, only three customers were actively using them.
From RAG to Deep Research: Building an Intelligent Agent
The 11x team knew they needed a different approach—something that would not just solve the technical problem but also build user confidence. Working with Letta, they developed what they called the "Alice Deep Research Agent" that could not only query their knowledge base (stored in Pinecone), but also synthesize information across documents and show customers exactly what information was being used by the agent. Letta agents show their reasoning steps, so this reasoning was shown to the customer to help them understand the agent’s behavior.
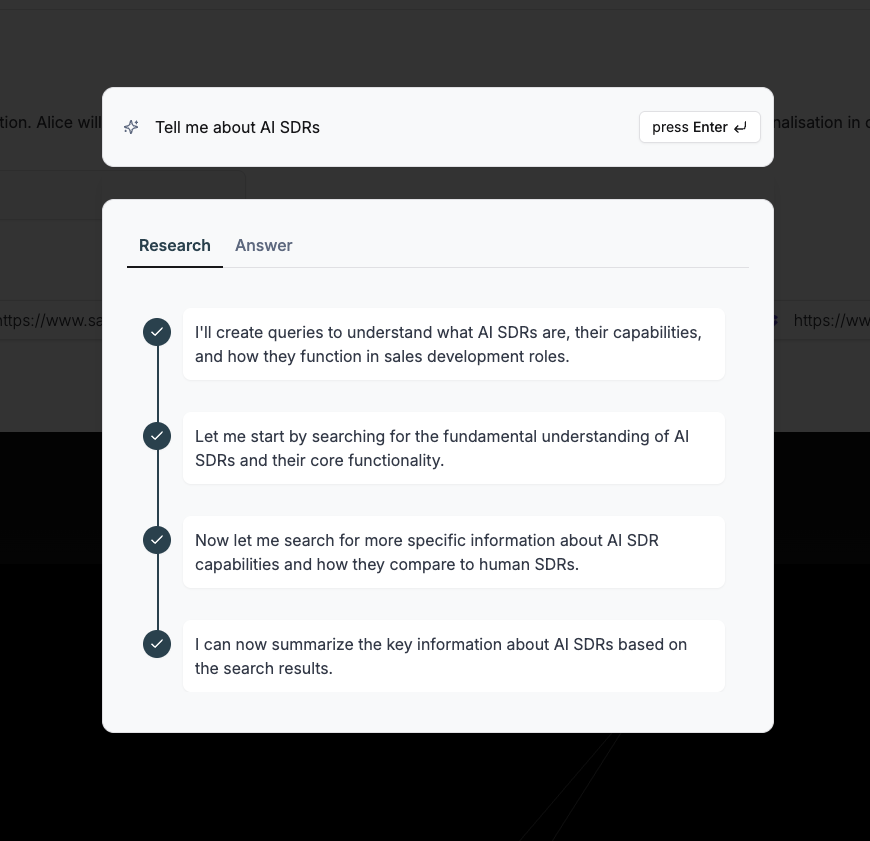

"The idea was that our agent should be able to intelligently read from data in our vast knowledge base," said Satwik Singh, an Engineer at 11x. Unlike a simple RAG system that would return chunks of text, the Deep Research agent actively processes and weighs different pieces of information through a process of iterative search (much like a human accessing a database), providing a cohesive, personalized response while showing its work along the way. The agent also transparently explains its reasoning at each step. Users can ask what the system knows about specific topics and watch the agent do research in real-time, building confidence in the AI's capabilities.
This level of transparency transforms how customers interact with the system. "If we did a simple RAG, we were putting the burden of forming the right query on the users," Satwik explained. "We don't want that. We want the users to just have a good experience, regardless of their level of understanding of how vector databases work."
Speed and Simplicity: Why Letta Made the Difference
For the 11x team, the most important factor that made Letta stand out compared to alternatives: development speed. "Speed was a huge factor. [Letta is] so easy to understand. Anyone can do it," said Fearon.
This dramatic acceleration in development matched 11x's need to iterate quickly. Satwik contrasted their experience with Letta against other frameworks: "When we're building agents there’s often this whole time period of making sure the graph's connected, the tool nodes are working... There's too much scaffolding. With Letta, there’s none of that. You jump into the ADE [Agent Development Environment], prototype as fast as possible, and it's a working solution."
The team initially planned to only prototypes in Letta’s ADE and deploy agents separately, but that transition never happened – instead, they stayed on Letta cloud even when moving to production. "We started just the prototype and then we were like, 'Well, this is pretty good. Why bother moving to when it's just gonna take us twice as long?'" Fearon explained.
Beyond speed, the 11x team found that Letta's approach to agent reliability through Tool Rules was significantly easier to implement than their previous experience with having to build explicit graphs. "I think it's a lot simpler than what we had to experiment with previously" Satwik noted. "With Letta, the tool rules were pretty obvious to set up."
From 3 Users to 85 Overnight
The impact of the Deep Research agent was immediate and dramatic. "We went from three customers who use it to now 85 overnight," revealed Fearon. This opt-in feature saw widespread adoption immediately after introduction.
“Our Account Executives and Sales Engineers are demoing it and loving it, customers as well.”, said Satwik. “The agent is loved across the board”.
The key to this transformation was addressing the trust barrier. By showing users exactly what information the system was using and where it came from, the team dramatically increased confidence in the AI capabilities. "It increases reply rates and user adoption, which we were struggling with actually before this. Now we have way more people," Fearon explained. The team is now tracking reply rates to measure the next level of impact.
Beyond the First Success: Building New Capabilities
What started as a solution to a context window problem has evolved into a foundation for new product capabilities. The team is now working on making their agents stateful – enabling them to learn over time what messaging works for different audiences.
Fearon painted a picture of how this could transform their product: "You learn what messaging works for what audiences over time and automatically improve your campaign. You kill a lot of manual A/B tests. It will automatically over time figure out and learn for each organization what's working and what's resonating." Beyond the immediate sales use case, the Deep Research agent has unlocked possibilities for entirely new product offerings. "This enables so many new products for us that we can build on top of this," explained Fearon. "It unlocks entirely new 11x workers longer term, like a marketing copywriter, SEO, blog writer, whatever we want to build."
One particularly promising direction for 11x is call recording analysis: "We're looking at building a call recorder where you can retrieve all of the context from the call. Imagine a solution engineer answering technical questions on the call. That would eliminate half of the headcount we're hiring for right now." By connecting their agents to CRM data, 11x is also exploring much more sophisticated sales targeting: "When you combine the CRM data, we're able to do super powerful things," Fearon noted. "Like 'find me all the people with closed-lost deals because we forgot to follow up a year ago.'"
What began as a technical solution to context window limitations has become a catalyst for reimagining what's possible in sales automation – replacing rigid structures with intelligent, transparent agents that can learn and adapt over time.
Try Letta today, or request a demo for your team.